This plan includes
- Limited free courses access
- Play & Pause Course Videos
- Video Recorded Lectures
- Learn on Mobile/PC/Tablet
- Quizzes and Real Projects
- Lifetime Course Certificate
- Email & Chat Support
What you'll learn?
- Machine Learning Core Concepts in Detail
- Understand use-case scenarios for applying Machine Learning
- Detailed coverage of Python for Data Science and Machine Learning
- Regression Algorithm - Linear Regression
- Classification Problems and Classification Algorithms
- Unsupervised Learning using K-Means Clustering
- Exploratory Data Analysis Techniques
- Dimensionality Reduction Techniques (PCA)
- Feature Engineering Techniques
- Model Optimization using Hyperparameter Tuning
- Model Optimization using Grid-Search Cross Validation
- Introduction to Deep Neural Networks
Course Overview
Pre-requisites
- Some exposure to Programming Languages will be useful
Target Audience
- Aspiring Machine Learning Engineers
- Aspiring Data Science Professionals
Curriculum 106 Lectures 21:25:04
Section 1 : Introduction to Machine Learning
- Lecture 2 :
- Machine Learning Terminology.
- Lecture 3 :
- History of Machine Learning
- Lecture 4 :
- Machine Learning Use Cases and Types
- Lecture 5 :
- Role of Data in Machine Learning
- Lecture 6 :
- Challenges in Machine Learning
- Lecture 7 :
- Machine Learning Life Cycle and Pipelines
- Lecture 8 :
- Regression Problems
- Lecture 9 :
- Regression Models and Perforance Metrics
- Lecture 10 :
- Classification Problems and Performance Metrics
- Lecture 11 :
- Optmizing Classificaton Metrics
- Lecture 12 :
- Bias and Variance
Section 2 : Statistical Techniques
- Lecture 1 :
- Types of Data and Descriptive Statistics
- Lecture 2 :
- Random Variables and Normal Distribution
- Lecture 3 :
- Histograms and Normal Approximation
- Lecture 4 :
- Central Limit Theorem
- Lecture 5 :
- Probability Theory
- Lecture 6 :
- Binomial Theory - Expected Value and Standard Error
- Lecture 7 :
- Hypothesis Testing
- Lecture 8 :
- Statistics and Experiments
Section 3 : Python for Data Science and Machine Learning
- Lecture 1 :
- Introduction to Python
- Lecture 2 :
- Starting with Python with Jupyter Notebook
- Lecture 3 :
- Python Variables and Conditions
- Lecture 4 :
- Python Iterations 1
- Lecture 5 :
- Python Iterations 2
- Lecture 6 :
- Python Lists
- Lecture 7 :
- Python Tuples
- Lecture 8 :
- Python Dictionaries 1
- Lecture 9 :
- Python Dictionaries 2
- Lecture 10 :
- Python Sets 1
- Lecture 11 :
- Python Sets 2
- Lecture 12 :
- Numpy Arrays 1
- Lecture 13 :
- Numpy Arrays 2
- Lecture 14 :
- Numpy Arrays 3
- Lecture 15 :
- Pandas Series 1
- Lecture 16 :
- Pandas Series 2
- Lecture 17 :
- Pandas Series 3
- Lecture 18 :
- Pandas Series 4
- Lecture 19 :
- Pandas DataFrame 1
- Lecture 20 :
- Pandas DataFrame 2
- Lecture 21 :
- Pandas DataFrame 3
- Lecture 22 :
- Pandas DataFrame 4
- Lecture 23 :
- Pandas DataFrame 5
- Lecture 24 :
- Pandas DataFrame 6
- Lecture 25 :
- Python User Defined Functions
- Lecture 26 :
- Python Lambda Functions
- Lecture 27 :
- Python Lambda Functions and Date-Time Operations
- Lecture 28 :
- Python String Operations
Section 4 : Exploratory Data Analysis
- Lecture 1 :
- Exploratory Data Analysis
- Lecture 2 :
- Tools and Processes of EDA
- Lecture 3 :
- EDA-Project-1
- Lecture 4 :
- EDA-Project-2
- Lecture 5 :
- EDA-Project-3
- Lecture 6 :
- EDA-Project-4
- Lecture 7 :
- EDA-Project-5
- Lecture 8 :
- EDA-Project-6
Section 5 : Linear Regression
- Lecture 1 :
- Linear Regression Introduction
- Lecture 2 :
- Training and Cost Function
- Lecture 3 :
- Cost Functions and Gradient Descent
- Lecture 4 :
- Linear Regression - Practical Approach
- Lecture 5 :
- Feature Scaling and Cost Functions
- Lecture 6 :
- OLS Assumptions and Testing
- Lecture 7 :
- Car Price Prediction
- Lecture 8 :
- Data Preparation and Analysis 1
- Lecture 9 :
- Data Preparation and Analysis 2
- Lecture 10 :
- Data Preparation and Analysis 3
- Lecture 11 :
- Model Building
- Lecture 12 :
- Model Evaluation and Optmization
- Lecture 13 :
- Model Optimization
Section 6 : Logistic Regression
- Lecture 1 :
- Logistic Regression Introduction
- Lecture 2 :
- Logit Model
- Lecture 3 :
- Telecom Churn Case Study
- Lecture 4 :
- Data Analysis and Feature Engineering
- Lecture 5 :
- Build the Logistic Model
- Lecture 6 :
- Model Evaluation - AUC-ROC
- Lecture 7 :
- Model Optimization
- Lecture 8 :
- Model Optimization
Section 7 : Naive Bayes Classification Algorithom
- Lecture 1 :
- Naive Bayes Probability Model
- Lecture 2 :
- Naive Bayes Probability Computation
- Lecture 3 :
- Employee Attrition Case Study
- Lecture 4 :
- Model Building and Optmization
Section 8 : Decision Tree Algorithm
- Lecture 1 :
- Decision Tree - Model Concept
- Lecture 2 :
- Decision Tree - Learning Steps
- Lecture 3 :
- Gini Index and Entropy Measures
- Lecture 4 :
- Pruning and Hyperparameter Tuning
- Lecture 5 :
- Iris Dataset Case Study
- Lecture 6 :
- Model Optimization using Grid Search Cross Validation
Section 9 : Random Forest Ensemble Algorithm
- Lecture 1 :
- Ensemble Techniques Bagging and Random Forest
- Lecture 2 :
- Random Forest Steps Pruning and Optimization
- Lecture 3 :
- Model Building and Hyperparameter Tuning using Grid Search CV
- Lecture 4 :
- Optimization Continued
Section 10 : Support Vector Machine
- Lecture 1 :
- Support Vector Machine Concepts
- Lecture 2 :
- Support Vector Machine Metrics and Polynomial SVM
- Lecture 3 :
- 84 Support Vector Machine Project 1
- Lecture 4 :
- Support Vector Machine Predictions
- Lecture 5 :
- Support Vector Machine - Classifying Polynomial Data
Section 11 : Dimensionality Reduction - Principle Component Analysis (PCA)
- Lecture 1 :
- Pricipal Component Analysis - Concepts
- Lecture 2 :
- Principal Component Analysis - Computations 1
- Lecture 3 :
- Principal Component Analysis - Computations 2
- Lecture 4 :
- Principal Component Analysis Practicals
Section 12 : Unsupervised Learning with K-Means Clustering
- Lecture 1 :
- Unsupervised Learning - K-Mean Clustering
- Lecture 2 :
- K-Means Clustering Computation
- Lecture 3 :
- K-Means Clustering Optimization
- Lecture 4 :
- K-Means - Data Preparation and Modelling
- Lecture 5 :
- K-Means - Model Optimization
Section 13 : Deep Learning
- Lecture 1 :
- Introduction to Deep Learning
Our learners work at
Frequently Asked Questions
How do i access the course after purchase?
It's simple. When you sign up, you'll immediately have unlimited viewing of thousands of expert courses, paths to guide your learning, tools to measure your skills and hands-on resources like exercise files. There’s no limit on what you can learn and you can cancel at any time.Are these video based online self-learning courses?
Yes. All of the courses comes with online video based lectures created by certified instructors. Instructors have crafted these courses with a blend of high quality interactive videos, lectures, quizzes & real world projects to give you an indepth knowledge about the topic.Can i play & pause the course as per my convenience?
Yes absolutely & thats one of the advantage of self-paced courses. You can anytime pause or resume the course & come back & forth from one lecture to another lecture, play the videos mulitple times & so on.How do i contact the instructor for any doubts or questions?
Most of these courses have general questions & answers already covered within the course lectures. However, if you need any further help from the instructor, you can use the inbuilt Chat with Instructor option to send a message to an instructor & they will reply you within 24 hours. You can ask as many questions as you want.Do i need a pc to access the course or can i do it on mobile & tablet as well?
Brilliant question? Isn't it? You can access the courses on any device like PC, Mobile, Tablet & even on a smart tv. For mobile & a tablet you can download the Learnfly android or an iOS app. If mobile app is not available in your country, you can access the course directly by visting our website, its fully mobile friendly.Do i get any certificate for the courses?
Yes. Once you complete any course on our platform along with provided assessments by the instructor, you will be eligble to get certificate of course completion.For how long can i access my course on the platform?
You require an active subscription to access courses on our platform. If your subscription is active, you can access any course on our platform with no restrictions.Is there any free trial?
Currently, we do not offer any free trial.Can i cancel anytime?
Yes, you can cancel your subscription at any time. Your subscription will auto-renew until you cancel, but why would you want to?
Instructor
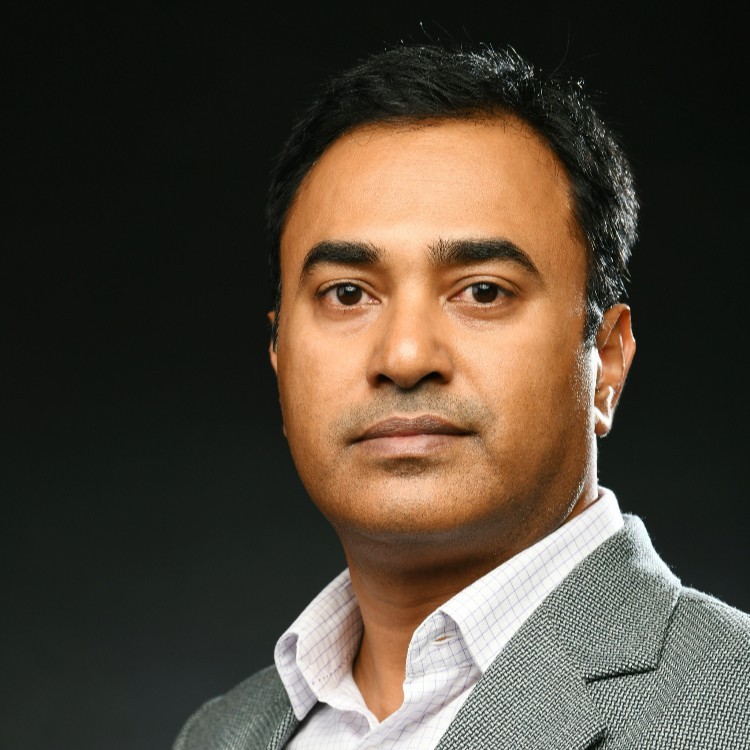
2263 Course Views
3 Courses